Revolutionizing High-Resolution Computer Vision: MIT is Breakthrough
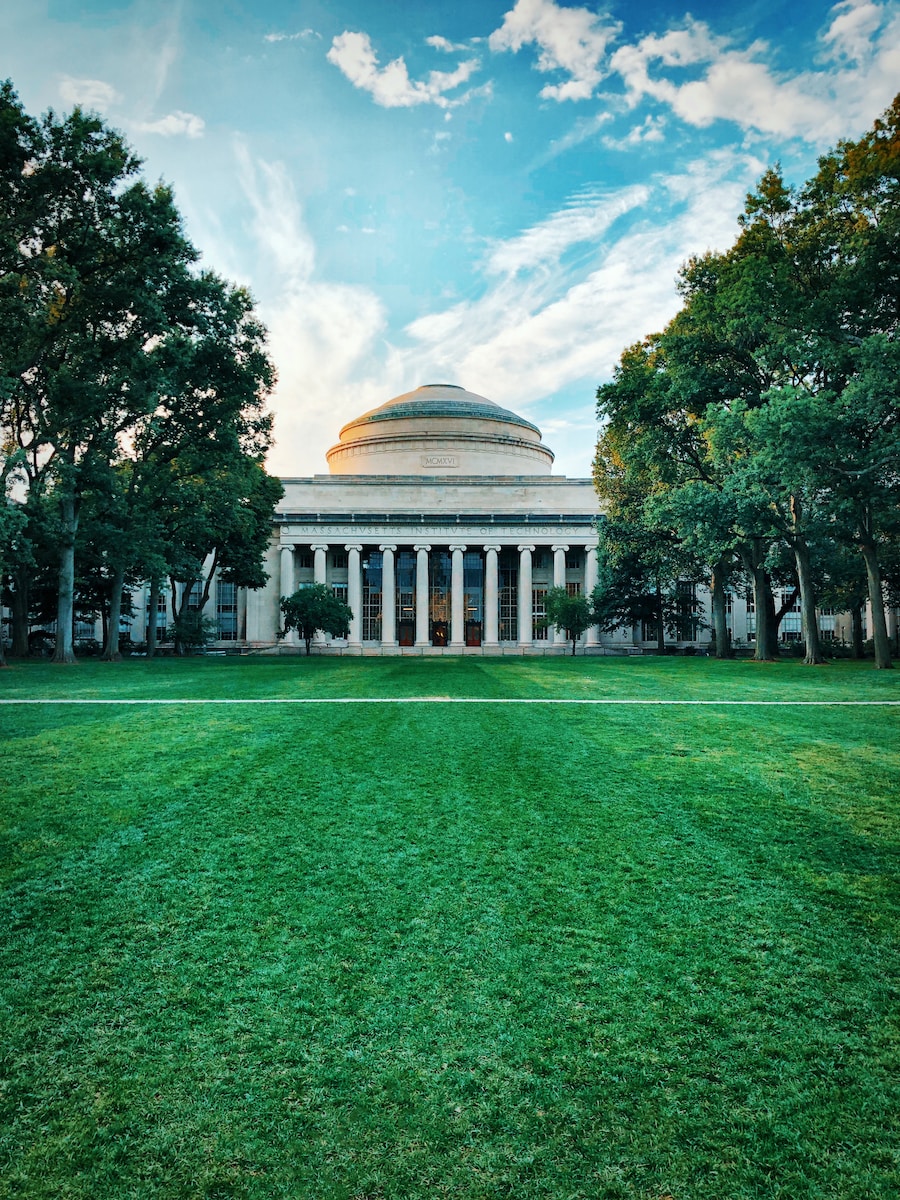
September 16, 2023
In the world of computer vision, speed and accuracy are essential, especially for tasks like object recognition in autonomous vehicles or enhancing image quality in video streaming. Traditional approaches to high-resolution image analysis often face computational challenges. However, researchers from MIT, in collaboration with the MIT-IBM Watson AI Lab and other institutions, have developed a groundbreaking solution that promises to transform the field.
The Need for Speed and Precision
Autonomous vehicles must swiftly identify objects, from parked delivery trucks to rapidly approaching cyclists. This task, known as semantic segmentation, involves categorizing every pixel in a high-resolution image. However, as image resolution increases, the computational complexity of this process grows exponentially.
Recent state-of-the-art semantic segmentation models, while accurate, struggle with processing high-resolution images in real time on edge devices like sensors or mobile phones. These models learn the interaction between each pair of pixels in an image, resulting in quadratic growth in calculations as image resolution increases.
How to Use Midjourney to Make an Infinite Zoom Video on an Image
EfficientViT: MIT Game-Changing Model
MIT’s researchers introduced an innovative solution in the form of EfficientViT, a new model series for high-resolution computer vision. EfficientViT achieves the same accuracy as state-of-the-art models but with only linear computational complexity and hardware-efficient operations.
The result is a model series that can perform up to nine times faster than previous models when deployed on mobile devices while maintaining or even improving accuracy. This breakthrough could have significant implications for real-time decision-making in autonomous vehicles and various high-resolution computer vision tasks, including medical image segmentation.
The Vision Transformer Concept
EfficientViT builds upon the concept of vision transformers, initially developed for natural language processing. These models divide an image into patches of pixels, encoding each patch into a token and generating an attention map. The attention map captures relationships between tokens, helping the model understand context.
However, traditional vision transformers’ attention maps grow exponentially with image resolution, causing computational challenges. In EfficientViT, MIT researchers simplified the attention map mechanism, replacing the nonlinear similarity function with a linear one. This adjustment allows for a linear growth in computation as image resolution increases.
To compensate for the potential loss in accuracy due to the linear function, two additional components were added to EfficientViT. One focuses on capturing local feature interactions, while the other facilitates multiscale learning to recognize both large and small objects. This balanced approach ensures both performance and efficiency.
Mastering AI: A Beginner’s Guide to Understanding and Utilizing Artificial Intelligence
A Hardware-Friendly Solution
EfficientViT’s design prioritizes hardware-friendliness, making it suitable for a range of devices, from autonomous vehicle computers to virtual reality headsets. This versatility extends its applicability to various computer vision tasks, including image classification.
Real-World Applications and Future Directions
EfficientViT’s performance improvements open doors to various applications, such as accelerating generative machine-learning models and further scaling it for other vision tasks. The model’s efficiency and capabilities are catching the attention of industry experts.
Lu Tian, senior director of AI algorithms at AMD, Inc., recognizes the potential of transformers in real-world applications, including enhancing image quality in video games. Jay Jackson, global vice president of artificial intelligence and machine learning at Oracle, acknowledges the significance of model compression and lightweight design for efficient AI computing.
MIT’s EfficientViT model series represents a significant breakthrough in high-resolution computer vision. Its ability to combine speed and precision while remaining hardware-friendly could pave the way for advancements in various fields, ultimately shaping the future of computer vision.
Official source of information: https://news.mit.edu/2023/ai-model-high-resolution-computer-vision-0912