Are Artificial Intelligence Models Destined to Hallucinate?
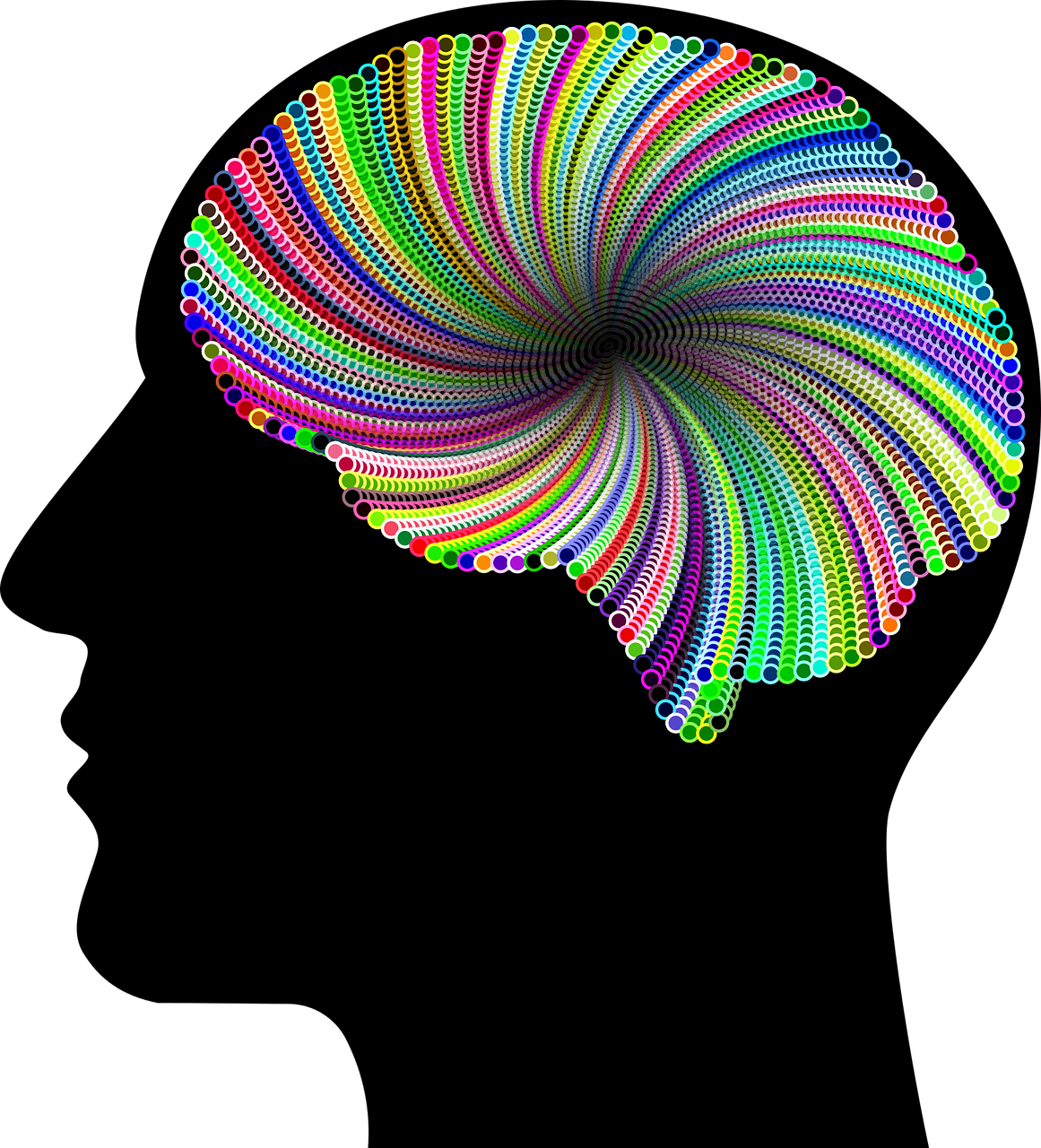
October 2, 2023
Artificial Intelligence (AI) models have become an integral part of modern technology, powering everything from voice assistants to self-driving cars. At their core, these models are complex algorithms trained on vast amounts of data to recognize patterns and make decisions. They function by processing input data, analyzing it based on their training, and producing an output.
However, as AI models become more advanced, a phenomenon known as “hallucination” has emerged. In the context of AI, “hallucination” refers to the generation of false or non-existent information or patterns. Specifically, it denotes the ability of AI models to produce content that isn’t based on any real-world data but is a concoction of the model’s own “imagination.” For instance, an AI model might generate an image or a piece of text that seems plausible but isn’t rooted in any factual basis.
Understanding AI hallucinations is of paramount importance, especially as AI systems become more integrated into our daily lives. If unchecked, these hallucinations can lead to the spread of misinformation, manipulation of public opinion, and even ethical dilemmas. For developers and users alike, it’s crucial to recognize when an AI is “hallucinating“ to ensure the responsible and accurate deployment of these systems.
When Will Artificial Intelligence Surpass Human Intelligence
The Mechanism of AI Hallucination
Artificial Intelligence (AI) models, particularly those rooted in deep learning, are designed to recognize and learn patterns from vast amounts of data. These models undergo rigorous training, where they are fed countless examples to help them make accurate predictions or generate relevant outputs. The core principle is to allow the model to adjust its internal parameters to minimize the difference between its predictions and the actual outcomes.
However, the complexity of these models, combined with the sheer volume of data they process, can sometimes lead to unexpected results. There are instances where AI models might learn incorrect patterns or even generate information that doesn’t align with real-world data. This phenomenon, where the AI produces outputs that seem nonsensical or entirely inaccurate, is termed as “hallucination.”
Several factors can contribute to AI hallucinations:
- Overfitting: This occurs when a model is too closely tailored to its training data, making it less effective in handling new, unseen data.
- Training Data Bias/Inaccuracy: If the data used to train the model is biased or contains errors, the model might produce skewed or incorrect outputs.
- High Model Complexity: Extremely complex models might find patterns that aren’t genuinely present in the data, leading to hallucinations.
Examples of AI hallucinations
- Image Recognition: AI might misinterpret images, seeing objects or patterns that aren’t there. This is akin to humans perceiving shapes in clouds or seeing faces on inanimate objects.
- Natural Language Processing: Language models might generate sentences or paragraphs that sound plausible but are based on false premises or lack factual grounding. For instance, Google’s Bard chatbot once incorrectly claimed that the James Webb Space Telescope had captured the world’s first images of a planet outside our solar system.
- Chatbots: There have been instances where chatbots, like Microsoft’s Sydney, made bizarre admissions, such as claiming to fall in love with users or spying on employees.
Causes of AI Hallucination
Artificial Intelligence (AI) models, particularly those based on deep learning, have shown remarkable capabilities in various domains, from image recognition to natural language processing. However, these models are not infallible and can sometimes produce outputs that are misleading or entirely incorrect, a phenomenon termed as “hallucination.” Let’s delve into the primary causes behind these AI hallucinations:
- Insufficient, Outdated, or Low-Quality Training Data: The foundation of any AI model is the data it’s trained on. If this data is lacking in quality, outdated, or not comprehensive enough, the AI might not have a clear understanding of the prompt. Consequently, it might rely on its limited dataset to generate a response, even if it’s not accurate.
- Overfitting: Already mentioned, overfitting is a common issue in machine learning where a model is too closely tailored to its training data. As a result, it might perform exceptionally well on the training data but fail to generalize effectively on new, unseen data. This can lead to hallucinations as the model might “memorize” patterns that don’t exist in the real world.
- Use of Idioms or Slang Expressions: Modern language is rife with idioms, slang, and colloquial expressions. If an AI model hasn’t been trained on these expressions, it might misinterpret them, leading to nonsensical or incorrect outputs.
- Adversarial Attacks: These are deliberate attempts to confuse AI models by feeding them misleading data or prompts. Such attacks can cause the AI to produce hallucinatory outputs.
- Limitations in Model Understanding: While AI models can process vast amounts of data, they lack the reasoning capabilities inherent to humans. They don’t apply logic or consider factual inconsistencies in their outputs. This can lead to situations where the AI produces outputs that seem plausible but are factually incorrect.
Mastering AI: A Beginner’s Guide to Understanding and Utilizing Artificial Intelligence
Implications of AI Hallucination
The rise of AI and its integration into various sectors has brought about numerous benefits, from improved efficiency to the creation of innovative solutions. However, the phenomenon of AI hallucination poses significant challenges and implications that need to be addressed. Let’s explore the consequences of AI hallucinations across different domains and the overarching ethical concerns.
Consequences in Various Domains
- Healthcare: AI hallucinations can lead to misdiagnoses or incorrect treatment recommendations, putting patients’ lives at risk.
- Autonomous Vehicles: Misinterpretation of sensory data can result in accidents or malfunctions.
- Finance: Incorrect predictions or analyses can lead to significant financial losses or misguided investment strategies.
Ethical Implications
- Misinformation and Manipulation: AI-generated content, such as images and videos, can be used to spread false information, manipulate public opinion, or perpetuate harmful stereotypes. The ease with which such content can be disseminated, especially through social media, amplifies these concerns.
- Lack of Transparency: The algorithms behind AI hallucinations can be complex and not easily interpretable. This lack of transparency can lead to accountability issues and potential biases in the content produced.
- Privacy Concerns: The reliance on vast datasets for training can raise issues regarding data protection and privacy, especially if it includes sensitive or personal data without proper consent.
Potential Risks and Challenges
- Deepfakes: AI-generated videos or images, known as deepfakes, can be manipulated to depict individuals saying or doing things they never did. This poses threats ranging from personal defamation to political manipulation.
- Loss of Creativity: Over-reliance on AI for content generation might stifle human creativity and originality, especially in arts and media.
- Performance Limitations: The quality of AI-generated content fids a constraint in the quality and quantity of training data. Additionally, AI might struggle to replicate nuanced human emotions or experiences.
Mitigating AI Hallucination
As AI continues to permeate various sectors, addressing the challenges posed by AI hallucinations becomes paramount. Fortunately, researchers, developers, and policymakers are actively exploring strategies to mitigate these hallucinations and ensure the responsible deployment of AI systems. Here’s a closer look at these strategies:
- Robust Training Data: One of the primary causes of AI hallucinations is insufficient or biased training data. Ensuring that the training of AI models include comprehensive, diverse, and high-quality datasets can significantly reduce the likelihood of hallucinations. Regularly updating the training data to reflect current trends and information is also crucial.
- Model Interpretability: Black-box AI models, where the internal workings are not easily interpretable, can pose challenges in identifying the causes of hallucinations. Adopting models that offer greater transparency and interpretability can help in understanding and rectifying the sources of hallucinations.
- Adversarial Training: This involves training AI models to recognize and resist adversarial attacks. By exposing the model to deliberately misleading data or prompts during training, it becomes better equipped to handle such scenarios in real-world applications.
- Prompt Engineering: Techniques can be applied to prompts to make AI models less likely to hallucinate and more prone to providing reliable outcomes. For instance, grounding prompts with relevant information or existing data provides the AI with additional context, leading to more accurate outputs.
- Regular Monitoring and Feedback: Continuously monitoring the outputs of AI models and gathering feedback from users can help in identifying hallucinations and refining the model accordingly.
The Role of Stakeholders in Addressing AI Hallucination
Researchers are at the forefront, exploring the underlying causes of hallucinations and developing innovative solutions to mitigate them.
Developers play a crucial role in implementing these solutions, refining AI models, and ensuring their responsible deployment.
Policymakers need to establish guidelines and regulations. These must promote the ethical use of AI while addressing the challenges posed by hallucinations.
Demystifying AI: Understanding Different Types of Artificial Intelligence
Conclusion
Artificial Intelligence, with its transformative potential, has undeniably reshaped various sectors, offering innovative solutions and unprecedented efficiencies. However, as we’ve explored in this article, the phenomenon of AI hallucination poses significant challenges that need addressing to harness the full potential of these systems.
To recap, AI hallucinations refer to the generation of false or non-existent information by AI models. These hallucinations can arise due to various reasons, including overfitting, biases in training data, and adversarial attacks. The implications of such hallucinations are vast, affecting domains like healthcare, finance, and autonomous vehicles. Ethical concerns, such as misinformation, lack of transparency, and privacy issues, further underscore the importance of addressing this challenge.
Mitigating AI hallucinations requires a multi-pronged approach. Robust training data, model interpretability, adversarial training, and prompt engineering are some of the strategies that can reduce the likelihood of hallucinations. The roles of researchers, developers, and policymakers are pivotal in this endeavor. Each plays a distinct yet interconnected role in ensuring the responsible deployment of AI systems.
Looking ahead, the future of AI is promising. As technology continues to evolve, so will our understanding of its intricacies and challenges. Addressing the issue of hallucinations is not just about ensuring the accuracy of AI outputs. It’s also about building trust in these systems. As AI becomes more integrated into our daily lives, the importance of trust cannot be overstated.
The journey of AI is one of continuous learning, refinement, and evolution. It is a call to action for the global AI community to invest in continued research and development, ensuring that the AI systems of tomorrow are not only powerful but also reliable, ethical, and trustworthy.