Demystifying AI: Understanding Different Types of Artificial Intelligence
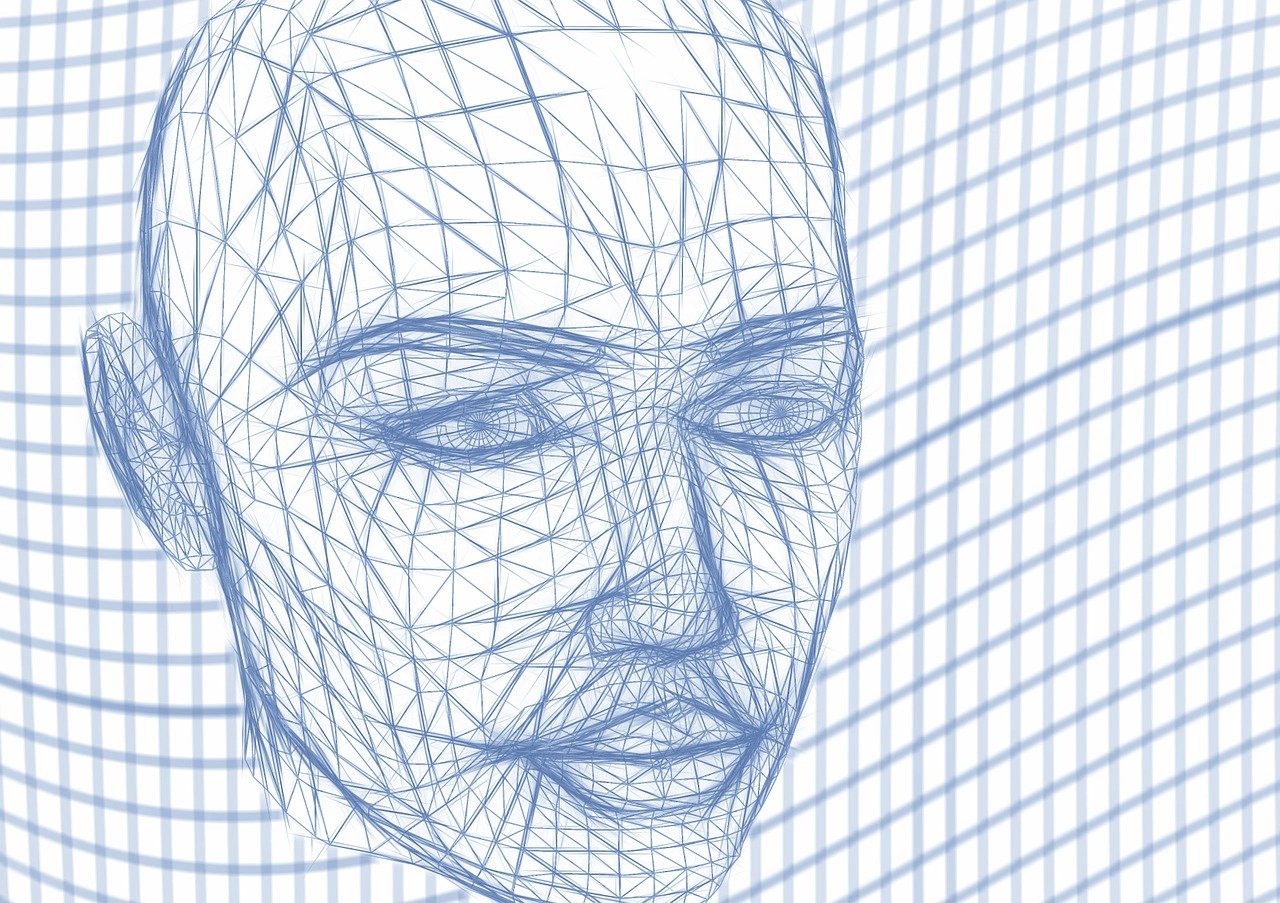
July 25, 2023
Artificial Intelligence (AI) has become a ubiquitous term in the 21st century, a buzzword that has permeated not just the technology industry, but also our everyday lives. From voice-activated virtual assistants to recommendation algorithms on our favorite streaming platforms, AI is increasingly shaping our interactions with the world around us. However, despite its prevalence, AI remains a nebulous concept for many. Often, it’s misunderstood or used as a catch-all term for any system that uses data to make decisions.
In reality, AI is a vast and complex field, encompassing a multitude of different types and subtypes, each with its own capabilities, uses, and implications. It’s a field that’s as diverse as it is dynamic, with new advancements and applications emerging at a rapid pace. For the uninitiated, this can make AI seem daunting and inaccessible, a labyrinth of jargon and concepts that are difficult to navigate.
How does artificial intelligence help us?
We can make it better
It doesn’t have to be this way. By breaking down AI, we can demystify this complex field and make it more accessible to everyone. Whether you’re a tech enthusiast looking to keep up with the latest developments, a business owner seeking to leverage AI in your operations, or a curious individual who simply wants to understand the technology that’s increasingly shaping our world, this article is for you.
In this article, we’ll peel back the layers of AI, exploring its different types and subtypes, and shedding light on what they mean and how they work. We’ll start by defining what AI is and what it isn’t, before delving into the distinctions between Narrow AI and General AI. We’ll then explore the various subtypes of AI, including Machine Learning, Deep Learning, and Reinforcement Learning, providing a clear and concise overview of these key concepts.
By the end of this article, you’ll have a solid understanding of the different types of AI, equipping you with the knowledge you need to navigate the world of AI with confidence. So, without further ado, let’s dive in and demystify AI.
Types of AI
Narrow AI (Weak AI)
Narrow AI, also known as weak AI, refers to AI systems that are designed to perform specific tasks or solve specific problems. These systems excel in a narrow domain but lack the ability to generalize beyond their predefined scope. Narrow AI applications are prevalent in our daily lives and have become integrated into many services and devices.
One common example of narrow AI is voice assistants such as Siri, Alexa, and Google Assistant. These intelligent assistants use natural language processing (NLP) and voice recognition algorithms to understand user commands and provide relevant information or perform requested tasks. They can answer questions, provide weather updates, set reminders, play music, control smart home devices, and much more.
Another example is streaming platforms like Netflix and Spotify, that use recommendation algorithms. These algorithms analyze user preferences, viewing or listening habits, and available content to suggest personalized recommendations, enhancing user experience and discovery of new content. They leverage techniques like collaborative filtering, content-based filtering, and machine learning to make accurate and relevant recommendations.
Image recognition systems, widely used in social media platforms, are another manifestation of narrow AI. These systems employ computer vision algorithms to identify and categorize objects, people, or scenes in images, facilitating content organization and search capabilities. Image recognition is also utilized in autonomous vehicles for detecting road signs, pedestrians, and obstacles.
General AI (Strong AI)
General AI, often referred to as strong AI, represents the concept of AI systems possessing human-like intelligence and the ability to understand, learn, and apply knowledge across a wide range of tasks and domains. While general AI remains a theoretical concept and has not yet been fully achieved, researchers and developers aspire to create machines capable of human-level cognition and reasoning.
Achieving general AI involves creating systems that can adapt to new situations, learn from experience, understand context, and perform tasks with flexibility and creativity. General AI would have a profound impact on various fields, including medicine, scientific research, and complex decision-making processes. However, achieving general AI raises ethical and philosophical questions, such as the potential impact on human employment and the boundaries of machine decision-making.
Machine Learning
Machine Learning (ML) is a subfield of Artificial Intelligence that focuses on enabling computers to learn from data and improve their performance without being explicitly programmed. ML algorithms can identify patterns and make predictions or decisions based on the data they receive.
- Supervised Learning: In supervised learning, algorithms learn from labeled data, where input-output pairs are provided during the training phase. For example, an email spam filter is trained on a dataset containing labeled spam and non-spam emails. By learning the patterns in the data, the algorithm can accurately classify incoming messages as spam or non-spam. Supervised learning algorithms include decision trees, support vector machines (SVM), and neural networks.
- Unsupervised Learning: Unsupervised learning algorithms work with unlabeled data, aiming to discover patterns or structures within the data. This approach is often used for tasks such as clustering, where similar data points are grouped together, or dimensionality reduction, which reduces the complexity of the data while preserving essential information. Popular unsupervised learning algorithms include k-means clustering, hierarchical clustering, and principal component analysis (PCA).
- Reinforcement Learning: Reinforcement learning involves training an agent to interact with an environment and learn optimal actions based on rewards and punishments. The agent receives feedback in the form of rewards or penalties as it navigates through the environment, enabling it to learn and improve its decision-making abilities. Game-playing AI, robotics, and autonomous vehicles commonly use reinforcement learning. Reinforcement learning scenarios see the use of Algorithms such as Q-learning and Deep Q-Networks (DQN).
Windows Copilot: Want to try Windows artificial intelligence now? Here’s how to do it
Deep Learning
Deep Learning (DL) is a subset of machine learning that focuses on artificial neural networks inspired by the structure and function of the human brain. Models, known as deep neural networks, consist of multiple layers of interconnected nodes called neurons.
Deep learning has achieved remarkable success in various domains, such as image recognition, natural language processing, and speech recognition. Its ability to automatically learn hierarchical representations from raw data makes it a powerful tool in AI research. Convolutional Neural Networks (CNNs), a type of deep learning architecture, are particularly effective in image and pattern recognition tasks.
Recurrent Neural Networks (RNNs) are suitable for processing sequential data, making them useful in tasks like language modeling and speech recognition. Transformers, a more recent development in deep learning, have proven highly effective for tasks involving sequence-to-sequence modeling, such as machine translation and natural language understanding.
Natural Language Processing (NLP)
Natural Language Processing is a branch of AI that deals with enabling computers to understand, interpret, and generate human language. NLP encompasses a wide range of tasks, including language translation, sentiment analysis, chatbots, and text summarization.
NLP techniques involve text parsing, which breaks down sentences into grammatical structures, allowing the computer to understand the relationships between words. Parts-of-speech tagging assigns grammatical labels to words, enabling the analysis of sentence structure. Named Entity Recognition (NER) identifies and classifies named entities like names, locations, and organizations within text.
Word embeddings, such as Word2Vec and GloVe, act to represent words as numerical vectors. They enable machines to grasp semantic relationships between words. Generating human-like text or predicting the next word in a sentence see the use of language modeling techniques, like recurrent neural networks (RNNs) or Transformers. Sentiment analysis algorithms aim to determine the emotional tone of a piece of text. The applications are for social media monitoring, customer feedback analysis, and opinion mining.
Computer Vision
Computer Vision focuses on enabling computers to understand and interpret visual information from images and videos. It involves tasks such as object detection, image classification, facial recognition, and image synthesis.
Computer vision algorithms employ various techniques, including feature extraction, where meaningful features are extracted from images to distinguish objects or patterns. Methods such as edge detection, scale-invariant feature transform (SIFT), or Histogram of Oriented Gradients (HOG) allow the extraction of features.
Computer vision tasks see the wide use of Convolutional Neural Networks (CNNs) due to their ability to automatically learn hierarchical representations from images. CNNs employ layers of convolutional filters to extract features at different levels of abstraction. These networks have achieved exceptional results in image classification, object detection, and image segmentation tasks.
Object detection algorithms use CNNs to identify and locate multiple objects within an image, often with bounding box annotations. Facial recognition systems employ computer vision techniques to recognize and authenticate individuals based on their facial features, enabling applications such as biometric security and identity verification.
My AI: Discover the Artificial Intelligence on Snapchat
A world of AI
Artificial Intelligence encompasses a diverse range of types and techniques, each with its unique applications and capabilities. From narrow AI systems designed for specific tasks to the theoretical concept of general AI, the field continues to evolve rapidly.
Machine learning, deep learning, natural language processing, and computer vision are among the key technologies driving advancements in AI. Understanding the different types of AI empowers us to comprehend their potential and limitations, paving the way for responsible and ethical deployment.
As AI continues to advance, it will undoubtedly shape the future of technology and society, offering new possibilities and challenges we have yet to imagine.